Abstract
This paper provides a comprehensive overview of COVID-19 related deaths within India over the first eight months of 2020 for two different Kaggle data sets. Analyzing first data set provided by the Kaggle for the period included Indian Nationality, states, and counts for total cases, deaths, and cured demonstrated that the states are statistically significant in a regression model.
Furthermore, the second Kaggle data set provided by the Kaggle for the period for age, gender, nationality, and all states in the country, I drew conclusions concerning correlations between COVID-19 deaths and the four factor categories and found that the overall logistics regression model was statistically significant. I concluded that within the first eight months of 2020, the both sexes are affected equally by the virus while age and states of residence play important roles in life and death due to the virus. Higher urban populated states with higher GDP creation have seen highest virus related deaths and may explain the forced avoidance of social distancing effect.
Introduction
India in 2019 with population of 1.37 billion and 0.73% death rate experienced about10 million deaths due to various causes. By end of August 2020 India has experienced more than 75,000 deaths due to COVID-19 virus infection cases of 3.3 million. [1]
Acharya et al. computed a composite index of vulnerability at the state and district levels based on 15 indicators across five domains of socioeconomic, demographic, housing and hygiene, epidemiological, and health system. They utilized a percentile ranking method to compute both domain-specific and overall vulnerability. They found that a number of districts in nine large states of Bihar, Madhya Pradesh, Telangana, Jharkhand, Uttar Pradesh, Maharashtra, West Bengal, Odisha, and Gujarat have high overall vulnerability with index value > 0·75. They observed similarities between vulnerability and the current concentration of COVID-19 cases at the state level but not at the district level.[2]
Das et al. documented the demographics and clinical profile of patients with ocular disorders presenting during the COVID-19 lockdown in India. This cross-sectional hospital-based four week study concluded that the enforcement of the nationwide lockdown resulted in a fewer overall patients presenting to the hospital from the local metropolitan region requiring about one fourth required a surgical intervention. Moreover there was an increasing trend seen in emergency patients from 46% in week 1 to 72% in week 4 and a decreasing trend in routine patients 45% in week1 to 21% in week 4. [3]
Mahajan et al., studied early part of demographic data (March-April 2020) and found that the median age of Indian COVID-19 patients was 39 which was quite lower than for China and Italy with the median age of 49.5 and 64 respectively. [4] [5] [6] The authors suggest that the difference in COVID-19 patient’s median age between the countries follows the fact that the median age for India is 28.4 years which is significantly lower than for China and Italy (38.4 and 41.9 years respectively) per UN population report. [7] Additionally the Indian study observed that the 76% cases were men of total confirmed cases which was quite different than for the Chinese and Italian studies where the ratio was roughly equal for men and women with an exception of South Korea where 60% women were found to be COVID-19 positive. [5] [6]
Kamath et al., list multiple challenges India faces for combating the COVID-19. It is densely populated (India: 464 people/km2 vs. USA: 36), has a huge population (India: 1,380 million vs. USA: 330 million), social distancing is not practical due to crowded streets, trains, buses and offices. Most people do not follow hygiene (e.g. covering mouth while coughing, washing hands with soap before eating) and millions do not have access to clean water for hand washing. [8] Moreover with a high prevalence of diabetes and hypertension for Indian adults which are known to have negative clinical outcomes with COVID-19. [9]
This paper specifically looks at demographic factors of age, gender, nationality, states of residence to understand statistically significant demographic factors that could clarify COVID-19 deaths in India. This comprehensive analysis is conducted over the first eight months of 2020 using two different Kaggle based data sets. Understanding of effects of the Indian demographic factors on COVID-19 deaths will guide us in developing better public policies to fight the pandemic.
Data acquisition and analysis
Database selection
I used data collected by Kaggle for both analyses, limiting the dataset to COVID-19 deaths for the first eight months for 2020. [10] [11] The first data set included 5,336 observations for nationality, states of residents, and counts for the total cases, deaths, and cured for COVID-19. [10] However, most of the data is missing nationality information.
I cannot make any conclusions concerning gender, age and COVID-19 deaths from the first data set as it did not include this information. [10] Therefore I turned again to Kaggle’s second data set for the second analysis which included factors of age, gender, nationality, states of residence, and their respective COVID-19 status (cured, hospitalized, dead) for a total of 28,182 observations. However, some of the data is missing age and gender information.
Hypothesis testing
Statistical analyses were performed with both data sets. I utilized a generic multiple regression testing model with interactions with independent variable y with xi as independent variables with i = 1,2,…
y=β0+β1×1+β2×2+….+ε ………….. (1)
The hypothesis used for the multiple regression model:
H0: β1=β2=…..=0 ……………… (2)
HA: at least one of βi≠0. …………………. (3)
For the nominal logistics regression model with independent variable y given by equation (4):
Iny1-y=β0+β1×1+β2×2+…… +ε …….. (4)
Rejection of H0 for the model implies that at least one of the independent variables contributes significantly to the model. The F-test I utilized to test the above hypothesis is with α = 0.05.
The hypothesis used for the multiple logistics regression model:
H0: β1=β2=….=0 ……………….. (5)
HA: at least one of βi≠0. …………. (6)
Rejection of H0 for the model implies that at least one of the independent variables contributes significantly to the model. The ChiSquare-test I utilized to test the above hypothesis is with α = 0.05.
Data analysis
First analysis model
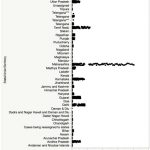
A visual display of the Kaggle-based first dataset is provided in Figure 1 for the first analysis model. The scatterplot shows variations between state/territory and COVID-19 caused deaths. It demonstrates that the state of Maharashtra has experienced more variation of deaths followed Tamil Nadu, Delhi, Karnataka, Gujarat, Andhra Pradesh, West Bengal, and Uttar Pradesh. The other states/territories have seen relatively lower deaths.
Using JMP software, I performed a linear regression analysis for COVID-19 caused deaths as a dependent variable and states/territories as independent variable. The model’s summary of fit demonstrated an R-squared adjusted value of 0.3732. The analysis of variance of the full model is shown in Table 1. The overall model is significant with a p-value of <0.0001. This means that the regression model is better than just using a mean value to predict deaths. Table 2 shows that all individual sources of states/territories are significant with COVID-19 deaths as it has a p-value <0.0001. Analyzing for the p-values I conclude that most states/territories are significant to the regression model with a p-value <0.05. A few states/territories (Andhra Pradesh, Dadra Nagar Haveli, Daman and Diu, Haryana, Madhya Pradesh, Panjab, Rajasthan, Telangana, Uttar Pradesh, and Unassigned) are not significant to the regression model with p-value >0.05.
ANOVA table – 1st data set
Source | DF | Sum of Squares | Mean Square | F Ratio |
Model | 41 | 4543842139 | 110825418 | 78.4799 |
Error | 5294 | 7475927678 | 1412151.1 | Prob>F |
C. Total | 5335 | 1.202e+10 | <0.0001 |
Effect tests – 1st data set
Source | DF | Sum of Squares | F Ratio | Prob>F |
State/Territory | 41 | 4543842139 | 78.4799 | <0.0001 |
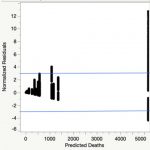
Figure 2 shows the normalized residuals vs. predicted deaths for the Kaggle data set 1 based model. There are many outliers when predicted deaths are higher than 5,000, as several of the points do not fall within a -3 to +3 range.
Second analysis model
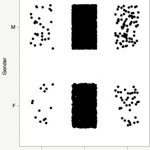
For the second model, multiple regression was performed using Kaggle’s second data set. The model included age, gender, states of residence, and count of COVID-19 deaths. A visual display of Kaggle-based second dataset is provided in Figure 3 through 6. These scatterplots show variations between gender and current status, age and current status, and states/territories and current status where current status takes a value of Deceased or Hospitalized or Recovered. Figure 3 demonstrates that both Males and Females experienced similar variations for each of the current status.
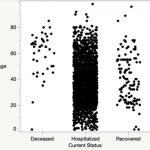
Figure 4 shows an increase in the number of deaths as a function of age. I reach the conclusion that if you are below the age of 40 your chance of death is very small while if you in the age group of 40 and above your chance of death is relatively higher. Most hospitalizations are for age of 70 and below while there are only few recoveries for the age over 70.
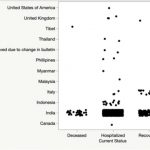
I have plotted nationality and current status related to COVID-19 based infections in Figure 5. It shows that Indian nationals are mostly affected on all fronts (diseased or hospitalized or recovered) compared to the foreign nationals being affected in the country.
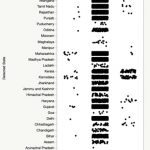
From Figure 6 , most states/territories have COVID-19 infected patients still hospitalized and in comparison the deaths are lot smaller in magnitude. The states of Kerala and Karnataka have most recovered patients than the other states in the country (diseased or hospitalized or recovered) compared to the foreign nationals being affected in the country.
I performed a multiple nominal logistics regression with current status as dependent variable y described by equation (4).Table 3 shows that the overall logistics regression model for the second Kaggle data set is significant with a p-value of <0.0001.
This means that the regression model is better than just using a mean value to predict deaths. The model had a R-squared value of 0.4105 Table 4 for this analysis demonstrated that age and states/territories of residence are statistically significant (p-value <0.05), however the gender is not statically significant with p-value of 0.0968 since it is greater than 0.05.
Whole model test – 2nd data set
Model | DF | -log likelihood | Chi Square | Prob>Chi Square |
Difference | 236 | 260 | 521 | <0.0001 |
Full | 374 | |||
Reduced | 634 |
Effect likelihood ratio tests – 2nd data
Source | DF | L-R ChiSq | Prob>ChiSq |
Age | 174 | 248.3 | <0.0002 |
Gender | 2 | 4.7 | 0.0968 |
Detected State | 60 | 260.7 | <0.0001 |
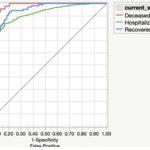
In Figure 7 I show the Receiver Operating Characteristics (ROC) plot for Kaggle’s second dataset which depicts sensitivity vs (1-specificity) for all three possibilities of current status due to virus infection. The area under the curve is the indicator of the goodness of fit for the model. A value of 1 indicating a perfect fit while value of 0.5 (represented by the diagonal in the figure) meaning that the model cannot discriminate among groups under consideration. Notice that all three curves have area values closer to 1 than 0.5 meaning a very good fit with the deceased group with the best fit out of all three options for the current status.
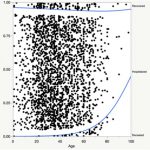
The second data set was run again for nominal logistics regression with this time age as the only independent variable and current status as a dependent variable. Figure 8 shows a logistics curve for this analysis. The lower curve in the plot shows the predictive probability of individual being diseased due to the virus depending on the age of the individual. The rising of the lower curve shows that higher the age higher the predictive probability of being diseased due to the virus. The upper curve shows the predictive probability of diseased or hospitalized individual on the basis of age. The distance between the two curves is the predictive probability of being hospitalized due to the virus depending on individual’s age. Thus higher the age relatively lower the predictive probability of being hospitalized compared to their younger counterparts. Notice also that the upper curve is flatter which means that combined predictive probability of diseased or hospitalized appears to be constant.
Understanding Indian population
Table 5 depicts India’s top populated states with ranking based on urban population. [12]
India’s top 10 populated states
State | Urban Rank | Urban Population | Total Population |
Maharashtra | 1 | 50.8M | 112.4M |
Uttar Pradesh | 2 | 44.5M | 199.8M |
Tamil Nadu | 3 | 34.9M | 72.1M |
West Bengal | 4 | 29.1M | 91.3M |
Gujarat | 5 | 25.7M | 60.4M |
Karnataka | 6 | 23.6M | 61.1M |
Madhya Pradesh | 7 | 20.1M | 72.6M |
Rajasthan | 8 | 17.0M | 68.5M |
Andhra Pradesh | 9 | 14.6M | 49.6M |
Bihar | 10 | 11.8M | 104.1M |
I created Table 6 with the COVID-19 death rankings derived from Figure 1. I also added GDP rankings for the states for 2019-2020. [13] COVID-19 deaths rankings for the states of residence match well with combination of urban population and GDP rankings. GDP creation requires people to interact with each other as close proximity realized in higher urban density setting would exacerbate the social distancing issues faced by the population.
India’s top 10 COVID 19 death states
State | Urban Rank | GDP Rank | COVID-19 Death Rank |
Maharashtra | 1 | 1 | 1 |
Uttar Pradesh | 2 | 5 | 6 |
Tamil Nadu | 3 | 2 | 2 |
West Bengal | 4 | 6 | 7 |
Gujarat | 5 | 3 | 4 |
Karnataka | 6 | 4 | 3 |
Madhya Pradesh | 7 | 11 | 8 |
Rajasthan | 8 | 8 | 9 |
Andhra Pradesh | 9 | 7 | 5 |
Bihar | 10 | 15 | 10 |
Conclusions
None.
Source of Funding
No financial support was received for the work within this manuscript.
Conflicts of Interests
None.
References
[2] R Acharya A Porwal A vulnerability index for the management of and response to the COVID-19 epidemic in India: an ecological studyLancet Global Health202089e11425110.1016/s2214-109x(20)30300-4
[3] A Das R Narayanan Demographics and clinical presentation of patients with ocular disorders during the COVID-19 lockdown in India: A reportIndian J Ophthalmol20206871393910.4103/ijo.ijo_1171_20
[4] P Mahajan J Kaushal Epidemic Trend of COVID-19 Transmission in India During Lockdown-1 PhaseJ Community Health20204561291130010.1007/s10900-020-00863-3
[5] H Shi X Han N Jiang Y Cao Radiological findings from 81 patients with COVID-19 pneumonia in Wuhan, China: A descriptive studyLancet Infect Dis202020442534
[6] E Livingston K Bucher Coronavirus Disease 2019 (COVID-19) in ItalyJAMA202032314133510.1001/jama.2020.4344
[7] Population Division World Prospects 2019. United Nations Department of Economics and Social Affairs, Population Division2019
[8] S Saaliq Limited clean water access in India spawns COVID-19 concerns2020
[9] S Kamath R Kamath P Salins COVID-19 pandemic in India: challenges and silver liningsPostgrad Med J2020961137422310.1136/postgradmedj-2020-137780
[10] Kaggle Dataset on novel Corona virus disease 2019 in India. covid_19_india.csv2020
[11] Kaggle Dataset on novel Corona virus disease 2019 in India2020
[12] Planning Commission, Government of India Census 2011-Demographic details, Literate Population2020
[13] India Reserve Bank of India. Handbook of Statistics on Indian StatesHandbook of Statistics on Indian States2016
1 comment
test